Can innovative statistical analysis support rare disease clinical trials?
27 February 2024 | By: Prof James Wason | 5 min read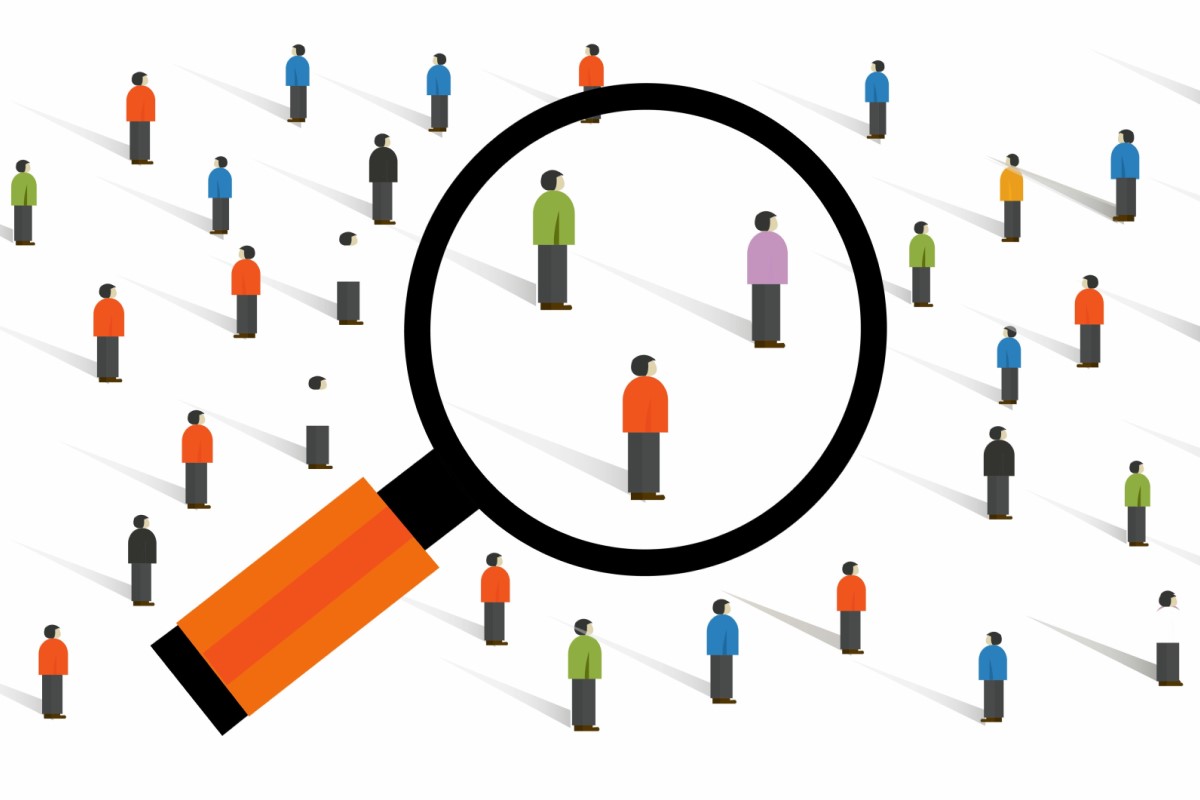
At Newcastle University we’ve been exploring new ways to design and analyse rare disease trials that result in good-quality evidence without needing as many participants.
In this blog, Prof James Wason shares three different approaches to making rare disease trials more feasible.
Contents
- Exploring new approaches to designing and analysing rare disease trials
- An example of the problem: Primary Biliary Cholanitis and OCA
- Making an impossible trial possible: synthetic control groups
- Making trials more feasible: reducing trial size, duration, and cost
- Running a rare disease trial within a basket trial
- Conclusions
Exploring new approaches to designing and analysing rare disease trials
In the UK a rare disease is defined as a condition that affects less than 1 in 2000 people. As there are many thousands of rare diseases, although each one is individually uncommon, it is estimated that 6-10% of people are affected by at least one.
Clinical trials are an essential part of showing new treatments are safe and effective, but they are really difficult to do for rare diseases. As a result, many patients suffering from rare diseases lack treatment options and hope.
The main cause of rare disease trials being difficult to do is self-explanatory: rare diseases affect fewer people. It is, therefore, more challenging to enrol the number of people needed for clinical trials using standard approaches.
At Newcastle University we’ve been exploring new approaches to designing and analysing rare disease trials that can result in good-quality evidence without requiring the numbers traditionally needed for trials.
This blog describes three different approaches to making rare disease trials more feasible:
- Using other sources of data to provide long-term evidence on the effectiveness of a drug
- Reducing the sample size required for trials with more efficient statistical analysis
- Adding a rare disease evaluation alongside more common disease using a basket trial design
An example of the problem: Primary Biliary Cholanitis and OCA
Primary Biliary Cholanitis (PBC) is a chronic autoimmune liver disease. It causes bile to build up in the liver, which damages it. Without treatment, patients will progress to needing a liver transplant or dying. It is a rare disease, with the prevalence estimated at around 0.04%. 90% of those diagnosed are women, normally between the ages of 40 and 60.
The first line treatment for PBC is Ursodeoxycholic acid (UDCA). Unfortunately, a third of patients have an inadequate response to UDCA. The second line therapy is obeticholic acid (OCA). It is more expensive and less well-tolerated than UDCA. But OCA has an interesting trial history.
Following a trial called POISE – the PBC OCA International Study of Efficacy – the US Federal Drug Administration had granted conditional approval for OCA in 2016. POISE provided convincing short-term evidence that OCA improved liver function. But the U.S Food and Drug Administration (FDA) requested long-term evidence of effectiveness for serious liver-related issues. Because participants in POISE were all given OCA after a year, the trial did not provide evidence about the long-term effect that the FDA requested.
The COBALT trial was set up to give randomised evidence on this. But with the drug conditionally approved, patients had less incentive to sign up for the trial owing to the chance that they would be allocated the placebo. Failure to recruit patients led to early termination of the COBALT trial and a strong risk of lacking the long-term evidence requested by regulators for full approval.
My team and I have explored different ways for innovative statistics techniques to reduce the risk of trials failing to recruit.
Making an impossible trial possible: synthetic control groups
With the COBALT trial unable to recruit enough patients to have its own control group, my team supported the creation of a synthetic control group1. In other words, could we use data from outside trials to provide the control group data and re-analyse the POISE trial data?
To form a robust synthetic control group we needed to use data that would allow a good match to the way the POISE trial had been completed. We needed data that would allow us to apply exclusion and inclusion criteria, review outcomes, and have patient characteristics data as close as possible to the POISE trial design.
We used two PBC research cohorts that had already run for several years, drawing from one UK and one global registry. This gave almost 14,000 patients from whom we could try to form synthetic control groups.
With suitable statistical analysis methods, we could account for differences between participants and registry data. The analysis we did provided high-quality evidence, that receiving OCA was associated with reducing the chance of dying or receiving a liver transplant compared to not receiving it.
Making trials more feasible: reducing trial size, duration and cost
Our second investigation considered the promotion of OCA to being a first-line therapy. If we could predict individuals with a high probability of non-response to the normal first-line therapy (UCDA), could patients benefit from OCA earlier?
We designed a randomised controlled trial2 for adults with recent onset PCB who were at enhanced risk of non-response to UDCA. The intervention would be OCA combined with UDCA for six months. The comparator group received a placebo combined with UDCA for six months.
The outcome to be investigated was binary: whether or not liver function was normal after six months of treatment. This is defined by checking whether two biomarkers - alkaline phosphatase (ALP) and bilirubin are within the normal range.
To detect increase in liver function normalisation from 20% to 45% with 90% power, the trial we designed would need to recruit for 36 months to reach 144 patients (more needed to cover dropouts), and would cost £2.1M. This was seen as too long and too much, so we looked for ways that we could reduce sample size, and therefore trial duration and cost.
Instead, we took an “augmented binary” approach whereby continuous components are considered alongside binary indicators. This is possible by first modelling the correlation between the continuous and binary components using a latent variable model.
The result of this approach was that we could:
- reduce the number of patients required for the trial from 144 to 106
- reduce the recruitment period by 12 months
- slash the required funding from £2.1M to £1.5M
The resulting trial, OPERA, was funded and is now underway.
Running a rare disease trial within a basket trial
Given that it is difficult to recruit people into rare disease trials, we wanted to explore if there is a way that we can undertake a trial of PBC alongside another trial for Parkinson’s Disease.
One of the symptoms in PBC is cognitive dysfunction such as short-term memory loss or deficits in attention. Although a distinct condition, Parkinson’s Disease also leads to similar cognitive dysfunction symptoms. There was substantial pre-clinical evidence that OCA might effectively treat cognitive symptoms.
We have designed the OACS trial3 to compare OCA and placebo impact in three participant groups:
- Patients with PBC who are non-cirrhotic but experience cognitive symptoms
- Patients with recent onset PBC with cognitive symptoms
- Patients with Parkinson’s Disease
To lend information across the different groups we use something called a commensurate prior approach. This allows us to study how consistent the results from the different groups appear to be. Where there is strong consistency, we can use this to inform further statistical analysis, leading to sharing information between the three trials and increased chance to show OCA is beneficial.
The OACS trial is still ongoing so there are no formal results yet. But our simulations show that this approach can increase the power and precision of the trial. We think this is a promising way to make more rare disease trials feasible – by conducting them within basket trials when a common drug shows promise for multiple conditions.
Conclusions
We're exploring three different statistical approaches to support rare disease clinical trials. They are synthetic control groups, correlating continuous indicators with binary data, and running rare disease trials inside larger basket trials. Our work shows that there is potential to decrease trial costs and complexity but still produce good quality evidence.
At Newcastle University we’re proud to be part of the community of researchers around the world looking to increase rare disease trial completion through innovative use of statistics. This has been a team effort with collaborations between clinicians, statisticians and trial experts.
Disclosures
1 Intercept Pharmaceuticals funded my team to create synthetic control groups from UK-PBC registry. No personal payments were received.
2 The randomised control trial used to investigate OCA as a first-line therapy was funded by the National Institute of Health and Care Research.
3 The OACS trial is an investigator-initiated study funded by Intercept Pharmaceuticals for which my team receives funding. No personal payments were received.
Selected references and further reading
- Borrowing of information across patient subgroups in a basket trial based on distributional discrepancy
- Bayesian sample size determination using commensurate priors to leverage pre-experimental data
You might also be interested in
- find out more about Prof James Wason, Professor of Biostatistics
- explore the Newcastle University Centre for Rare Disease and our long history of research and clinical care in rare disease
- visit the Newcastle University Population Health Sciences Institute website
- read about the Newcastle Joint Research Office - a partnership between The Newcastle upon Tyne Hospitals NHS Foundation Trust and Newcastle University, supporting the development, implementation, and delivery of world-class experimental, translational and clinical research